30
Sep 2024
AI Passport for Biomedical Research
Fundamentals of Biomedical AI Research
AI Passport for Biomedical Research is an accessible training program designed for healthcare professionals and biomedical researchers to integrate AI into their research and practice. With minimal to no coding, it caters to all technical levels using real-world medical data and case studies. The community-based learning program offers flexible asynchronous learning, live community sessions, and mentorship from AI faculty, coaches, and your fellow peers.
Explore the fundamentals of biomedical AI research and demystify artificial intelligence, while learning about its life cycle, designing biomedical experiments with AI, and how to train, validate and generalize AI in your research!
Course Outcomes
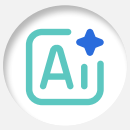
Integration of AI Techniques:
Participants will be able to integrate AI and machine learning techniques into their healthcare research and clinical practice.
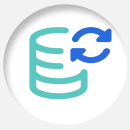
Application of Real-World Data:
Learners will effectively use real-world medical data and case studies to address healthcare challenges and develop innovative solutions.
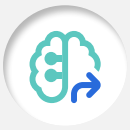
Practical AI Skills:
Graduates will gain hands-on experience with code-free AI/ML tools, enabling them to implement AI solutions without extensive programming knowledge.
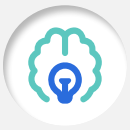
Critical Thinking and Problem Solving:
Participants will enhance their ability to analyze and solve complex healthcare problems through AI-driven insights and methodologies.
Module 1: Fundamentals of Biomedical AI Research
Understand the capabilities, limitations, and applications of biomedical AI and AI's lifecycle
Microskill:
- Demystifying artificial intelligence
- Artificial intelligence lifecycle
- Designing biomedical artificial intelligence experiments
- Training, validation, and generalizability
- Leveraging multidisciplinary team strengths
- Basics of scientific rigor and reproducibility
- Mentorship and peer review in biomedical AI
Module 2: Biomedical AI Alignment
Explore the ethics of biomedical AI, including bias, fairness, liability, and societal impact
Microskill :
- Fundamentals of biomedical AI ethics and liability
- Bias, fairness, and societal impact of biomedical AI
- The regulatory landscape of biomedical AI
- Biomedical AI quality and safety
- Human-AI collaboration in biomedicine
- Human-AI collaboration in biomedicine
- The fundamental principles of bioethics
Module 3: Data-Centric Biomedical AI
Explore the principles of developing an AI-ready biomedical dataset
Microskill:
- The importance of data for developing biomedical AI
- Acquiring ethically sourced biomedical data
- Understanding the role of human annotation
- Promoting FAIR biomedical data principles
- Developing AI/ML-ready biomedical datasets
- Navigating multi-institutional data sharing challenges
- Secure and ethical use of biomedical data
Module 4: Fundamentals of Biomedical ML
Evaluate the basics of machine learning and how to choose the right ML/deep learning model
Microskill:
- Shared biomedical artificial intelligence vocabulary
- Applied fundamentals of ML and deep learning
- Choosing the right biomedical machine learning model
- Choosing the right biomedical deep learning model
- Evaluating biomedical machine learning models
- Model generalizability
- Ethics of black-box algorithms
Module 5: Biomedical Image Analysis
Learn about the process and applications of biomedical AI image analysis
Microskill:
- Landscape of biomedical imaging
- Biomedical image preprocessing and transformation
- Traditional biomedical image analysis
- Biomedical computer vision applications
- Advanced and emerging topics
- Consistency in biomedical image analysis
- Ethical and privacy implications of biomedical imaging
Module 6: Generative AI in Biomedicine
Understand the fundamentals of generative biomedical AI and large language models (LLMs)
Microskill:
- Fundamentals of generative biomedical AI
- Fundamentals of large language models
- Large language models (LLMs) in biomedicine
- Prompt engineering for biomedical applications
- Utilizing LLMs for accelerating biomedical research
- Evaluation and reproducibility of AI-generated data
- Ethical dissemination of generated biomedical content
Module 7: Impact Project
Learn to design biomedical AI experiments, write successful proposals, effectively communicate research, and incorporate biomedical AI into traditional research
Microskill:
- Designing biomedical AI experiments
- Writing successful biomedical AI proposals
- Effective scientific communication
- Bridging traditional research with AI innovation
- Peer review and feedback mechanisms
- Robust biomedical AI research design
- Responsible biomedical AI research
Module 8: Agentic AI
Microskill:
- Designing biomedical AI experiments
- Writing successful biomedical AI proposals
- Effective scientific communication
- Bridging traditional research with AI innovation
- Peer review and feedback mechanisms
- Robust biomedical AI research design
- Responsible biomedical AI research
Meet Your Instructors
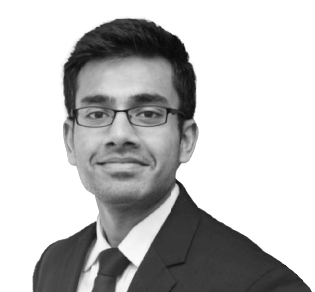
Ashish Aggarwal PhD
Instructional Associate Professor
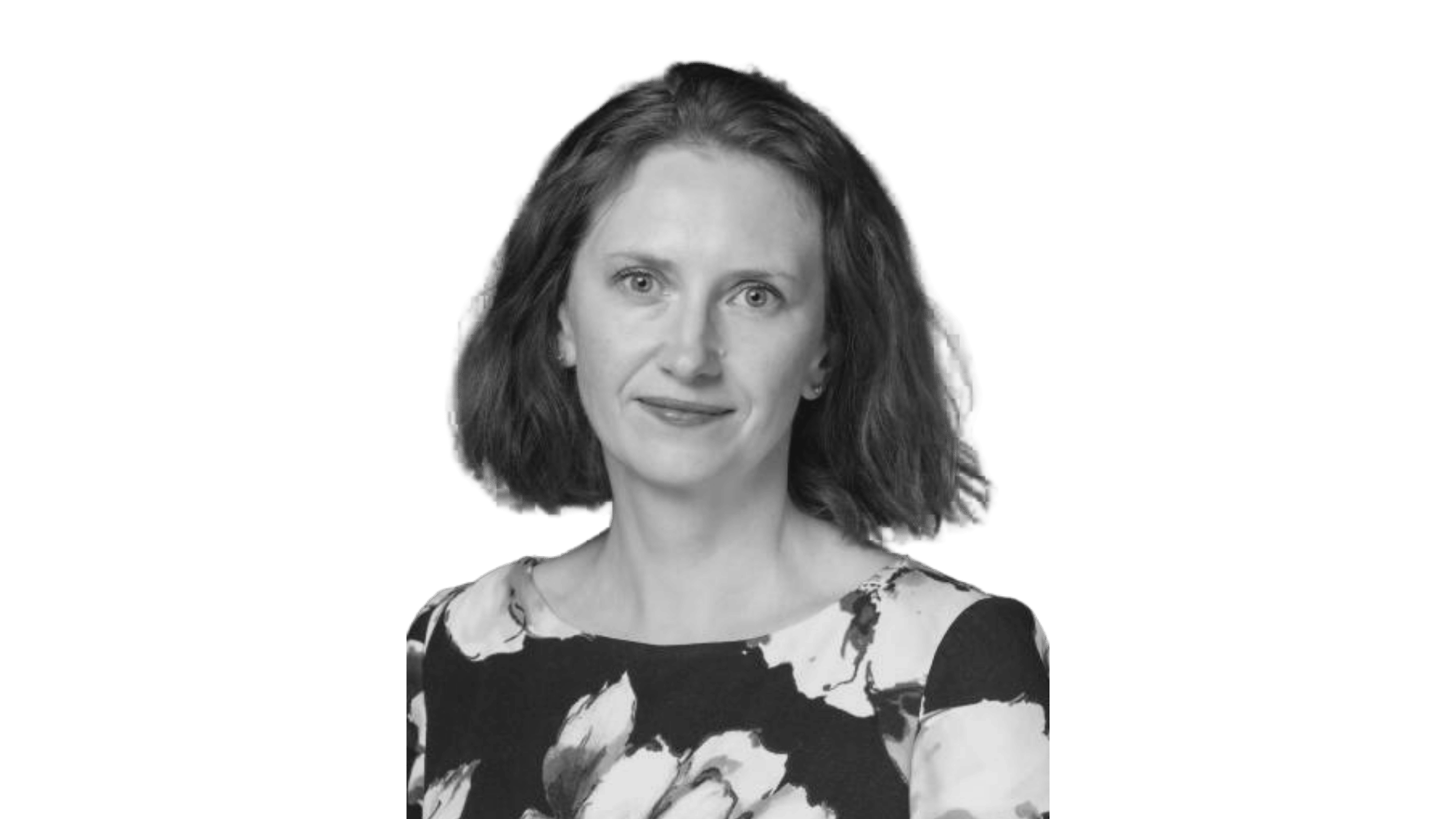
Yulia A. Levites PhD
Assistant Professor of Health Services Research, Management & Policy
Director of Evaluation and Educational Development of UF Clinical Transnational Science Institute
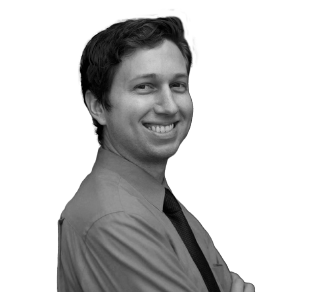
Benjamin Shickel PhD
Assistant Professor
Associate Director, Intelligent Clinical
Care Center
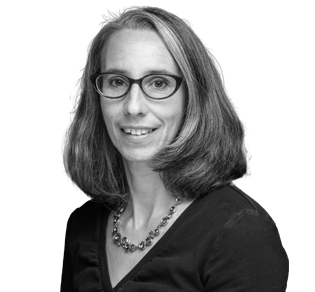
Elizabeth Palmer PhD
Assistant Director of Training and Education
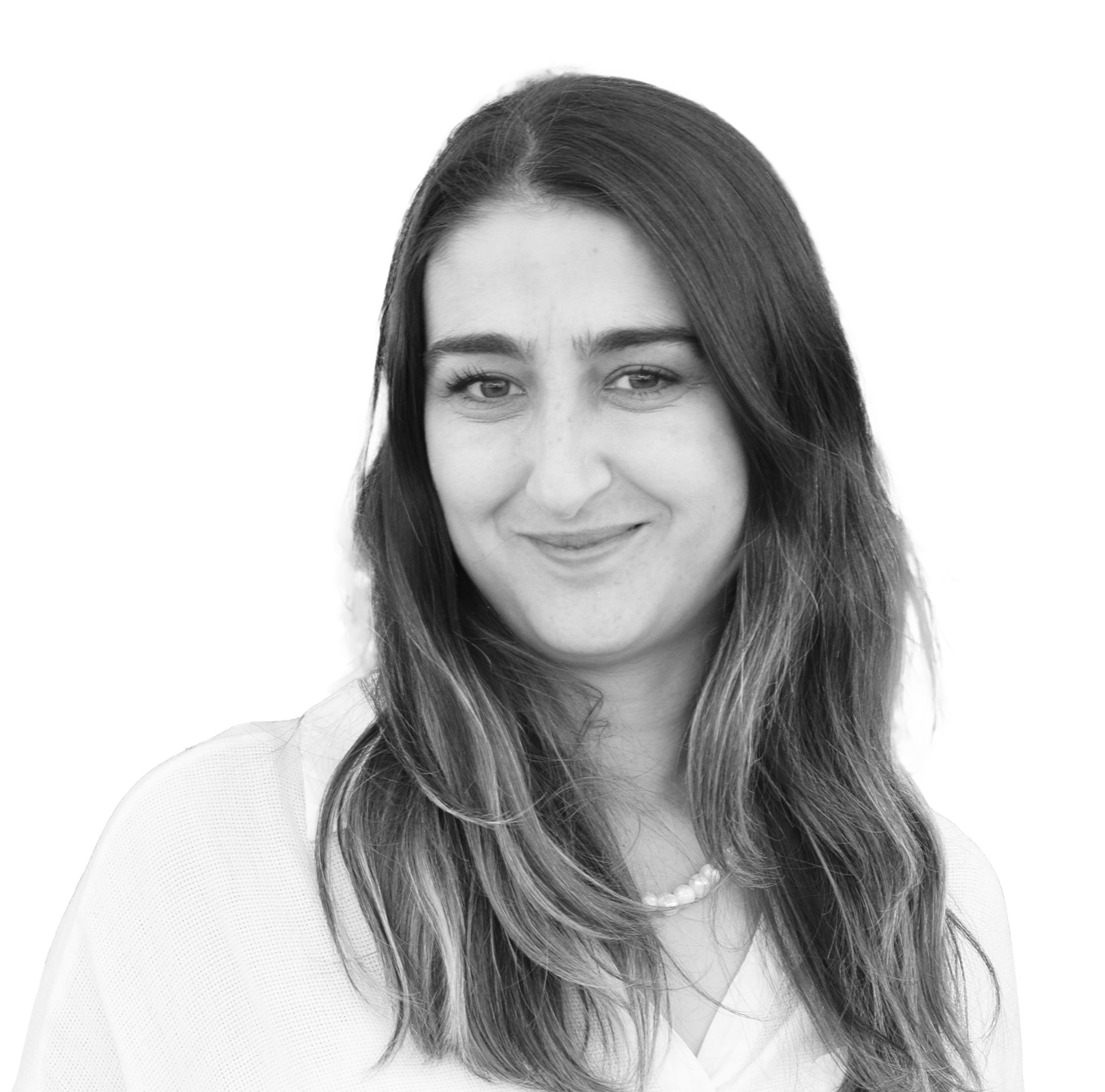
Tezcan Ozrazgat-Baslanti PhD
Associate Professor, Department of Medicine, UF College of Medicine
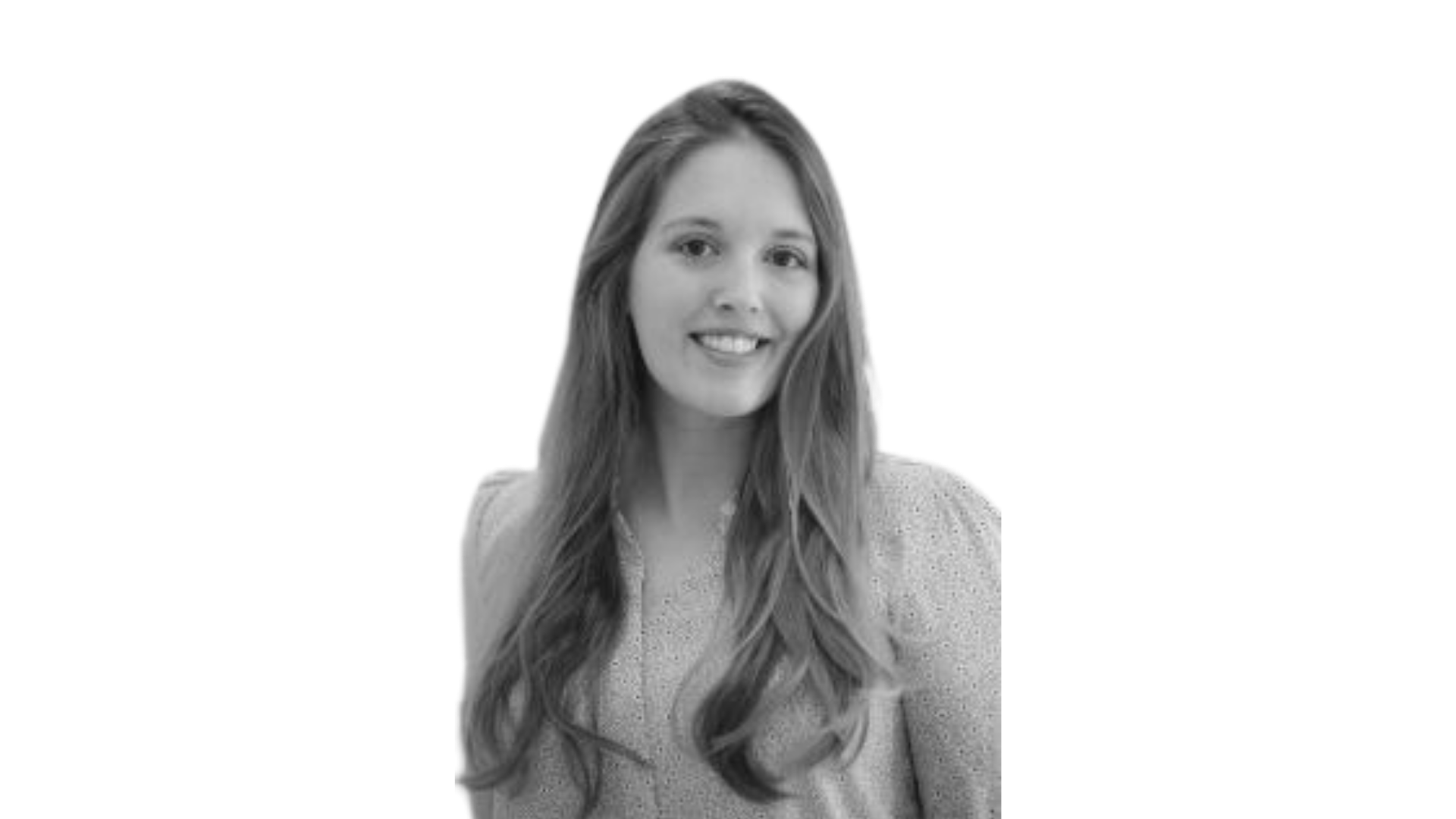
Rhonda Bacher PhD
Assistant Professor, Department of Biostatistics, College of Public Health and Health Professions & College of Medicine
Associate Professor of Surgery
Associate Director, Intelligent Clinical
Care Center
General Surgery Resident